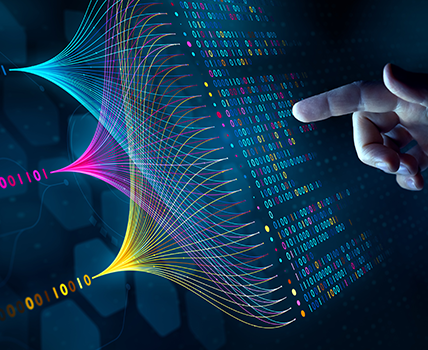
With all the hoopla surrounding artificial intelligence and machine learning (AI/ML), is it possible that only 10% of buy-side trading desks are incorporating these technologies in their equity trading processes?
Is this a case of “don’t believe the hype” or simply a longer adoption period than expected? Coalition Greenwich research has shown that more than 85% of asset managers have already implemented AI in some capacity for research and idea generation, so we were surprised to find that among the 90 buy-side traders we spoke with across the U.S. and Europe:
Our first reaction to the results was disbelief. How could almost three-quarters of equity traders not be planning to incorporate it? Maybe the responding traders didn’t know (or want to admit?) that their quant and data science teams are already moving forward?
Looking deeper into the data, of that 26% already incorporating AI/ML or planning to do so in next 12–24 months, the top three areas of AI/ML incorporation are:
As we noted in a recent study, AI, and especially ML, are often misunderstood. In many cases, rules-based programming and other types of models are responsible for analytical and predictive outcomes, which is not true for AI/ML. As a result, some respondents might have misperceptions, and the percentage of early adopters or those with plans to adopt may be even lower. In fact, a head of workflow technology went as far as saying, “I don’t think that more than 20% [of traders] understand what they mean when they claim to be using AI or ML.”
To help shed light on this dilemma, we spoke with several leading minds at asset managers, brokers and fintech providers.
One astute quantitative trading analyst noted, “I think there is some trepidation in the industry about calling anything AI, given the SEC’s proposals around data analytics and the October executive order on AI. It’s kind of scary, we don’t know what the scope will be.” They added that while the AI order was written with a focus on retail trading, institutions don’t yet know if it will apply to them. “The SEC has a closed-door policy; they’re not taking meetings on this. Are they actually reading comment letters?” they wondered.
A quant researcher/trading head guesses the 74% who are not planning on incorporating AI/ML are interpreting the question to refer to specific tools like ChatGPT, which they find “problematic to use” (non-disclosure of code, inaccuracy and unaccountability in results, etc.). However, they note that “There is no clean mathematical or scientific separation between the approaches behind large language models and more run-of-the-mill ML. Nor is there any shortage of low-hanging fruit where basic ML done well can yield better results.”
While we were not surprised that real-time algo trading optimization is a top area of AI/ML incorporation, one sell-side quantitative head we spoke with suggested there may be an element of response bias at play. People are inclined to improve tasks and functions they are responsible for over others they are not. Almost 75% of this study’s participants were head traders, who are generally most focused on trade execution. The more tech-savvy heads may also be indicating where they think the inherent noise is in the process, or the unwanted behavior and random or irrelevant variations or errors in the data that makes it more suitable for ML applications.
“A lot of effort goes into managing the noise,” a buy-side systematic trading head observed. The same trading analyst was surprised that real-time algo optimization ranked so high, noting that some uses of ML in this area are more expensive than others from a data perspective. “Unless you have an engine that can handle real-time, ticking data, I’m a little curious about how one does real-time optimization,” they added.
Another head of systematic trading also expected even lower overall usage than we found. For example, they wonder how many managers who claim to incorporate AI/ML into their real-time algo trading optimization are talking about using sell-side algorithmic strategies that leverage elements such as volume forecasting, limit order placement models and other features. In this case, managers could say they are using AI/ML in their trading process, but it is really the sell side that is doing the heavy lifting of incorporation.
This is an important distinction—much of what the buy side is leveraging at this point is probably provided by the sell side or a vendor, not proprietarily built in-house. “It’s not necessarily wrong,” the systematic trading head added, but could use “an added layer of nuance” around what it means to make use of such advanced technologies. Several others shared this notion.
One workflow solutions specialist was also curious if the respondents were including their use of broker or fintech vendor-derived models. “I’d think most of the Street could answer “yes” to this question if the models aren’t built in house,” they noted. Another thinks the traders may be claiming incorporation based on the pervasiveness of AI/ML claims made by their technology platform providers, for example in pre- or post-trade risk evaluation. “I suspect the true usage and tangible benefits are a small fraction of what’s reported.”
Others suggested perhaps some claims of AI/ML use in trade optimization are a bit “embellished” or “exaggerated.” For example, is simply clustering (classifying) stocks that share similar characteristics “using AI/ML in trading”? Technically it is, although several people felt that designation is best reserved for tasks like real-time decision-making. “What people mean by using AI/ML spans a pretty big range,” one person added.
The quant researcher we spoke with shared that sentiment, suggesting we be more specific in our questions. “AI/ML” has become a blanket term for an extensive mix of technologies, from basic automation tools like algo wheels to large language models like ChatGPT. “I would have to know how people interpreted this question for these answers to mean anything,” they noted.
For starters, perhaps we’ll revisit how we’ll pose such questions in future outreach. We’ll ask about AI and ML separately as the distinct technologies they represent. It’s clear that we should further distinguish between proprietary AI and ML models versus those leveraged via third parties, such as brokers and fintech providers. While one is not inherently better than the other, there’s a difference in how they are used. Besides, as our recent research has shown, many managers are now taking a hybrid “buy, build and integrate” approach to technology solutions.
For now, we’ll take this feedback constructively. ML is an iterative process that involves gathering and analyzing data to tune a model and make systematic improvements. Maybe we can take that same mindset and learn something not by iteratively asking the machines, but by iteratively asking about them.
Jesse Forster is the author of this publication.